DATE
26th April 2025
CATEGORY
Artificial Intelligence, IT Operations
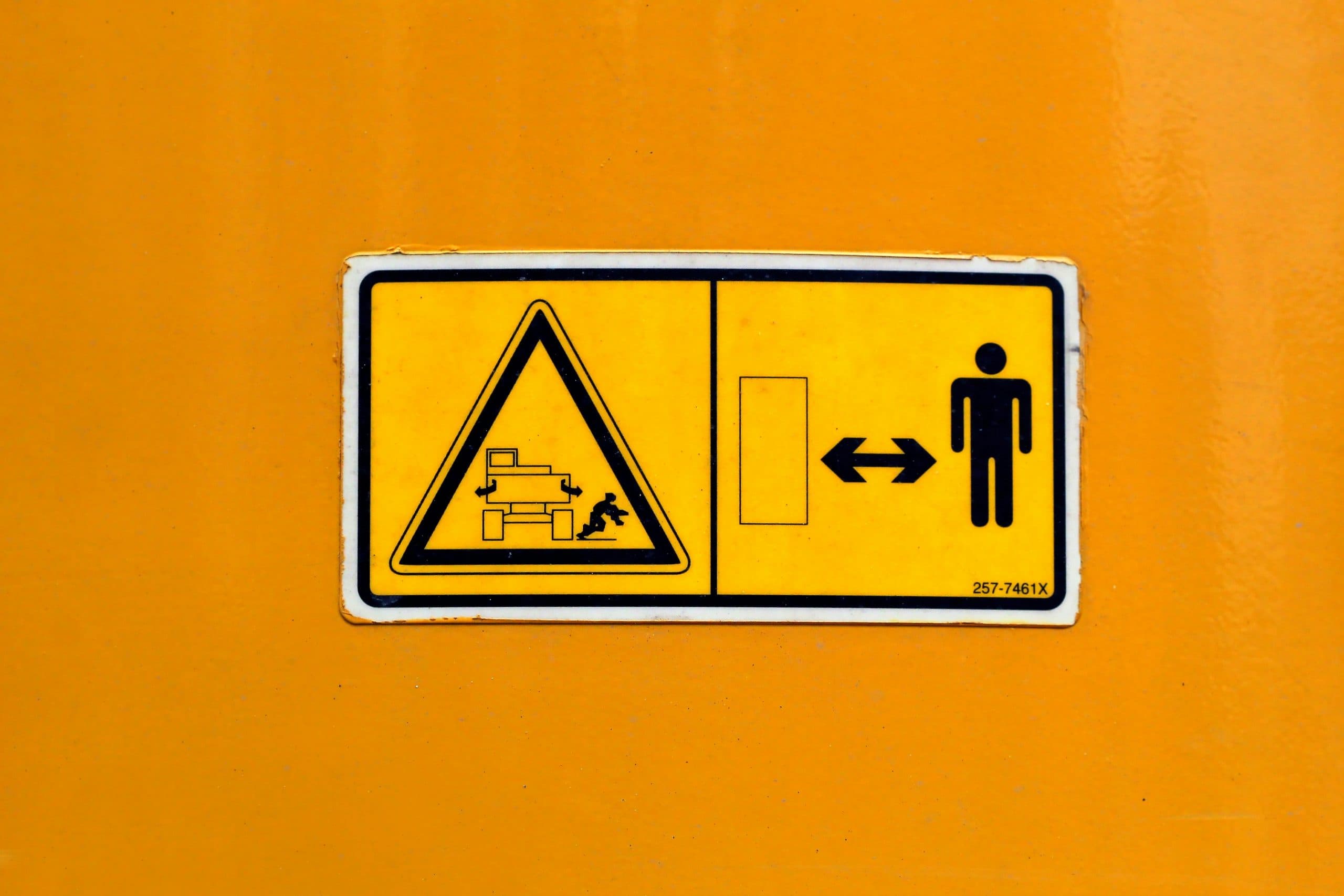
Understanding AI-Powered Risk Management
AI, or Artificial Intelligence, refers to the simulation of human intelligence processes by machines, particularly computer systems. In a business context, risk management encompasses the identification, assessment, and prioritization of risks followed by coordinated efforts to minimize, monitor, and control the probability of unfortunate events.
Historically, risk management practices relied heavily on past experiences, judgment, and traditional data analysis. However, the introduction of AI has revolutionized these practices, bringing much-needed precision and speed. AI tools can analyze vast amounts of data in real time, allowing businesses to anticipate risk scenarios that human analysts might not perceive.
By incorporating machine learning, automation, and predictive analytics, AI enhances traditional methodologies, offering more robust frameworks for managing risk. AI not only predicts risks but also offers tailored solutions based on shifting patterns.
The key benefits of integrating AI into risk management include improved accuracy in risk identification, faster decision-making processes, and more efficient resource allocation. AI systems can continuously learn from new data, ensuring that organizations are not just reacting to current risks but anticipating future challenges.
Pro Tip: Start small by implementing AI tools in specific areas of risk management to measure effectiveness before scaling to wider applications.
The Role of AI in Identifying Business Risks
Several AI technologies, such as machine learning and natural language processing (NLP), play pivotal roles in risk identification. Machine learning algorithms can learn from historical data patterns, while NLP can scour through documents and communications to identify potential risks from unstructured data.
AI’s capability to analyze historical data is fundamental in predicting potential risks. By examining trends over time and identifying anomalies, AI provides a predictive edge that traditional methods cannot match. Predictive analytics models can forecast risks before they evolve into significant issues, allowing for earlier intervention.
Real-world case studies demonstrate AI’s power in risk identification. For instance, financial institutions utilize AI-driven models to flag unusual transaction activities, catching fraudulent behavior often missed by manual processes. Additionally, insurance companies employ predictive analytics to assess risk profiles more effectively.
Pro Tip: Encourage the use of AI-driven platforms that allow you to visualize data, making it easier to assess potential risks and communicate findings across teams.
Mitigation Strategies: How AI Can Help
One significant advantage of AI is its capacity to automate risk response strategies. By using AI systems, organizations can implement protocols that automatically trigger specific responses when predefined risk thresholds are met.
Furthermore, AI optimizes resource allocation for risk mitigation activities. By analyzing data on past risk events, AI suggests where resources are most needed and where they can be most effective.
Scenario analysis and simulation models driven by AI empower businesses to prepare for multiple risk scenarios. By simulating various situations, organizations can create dynamic strategies that adapt to changing conditions—enhancing decision-making during critical risk intervention processes.
Pro Tip: Develop a playbook with AI-generated scenarios and automated responses to ensure swift actions in the face of emerging risks.
Implementing AI Solutions for Risk Management
Integrating AI into existing risk management frameworks involves several systematic steps. Organizations should begin by understanding their current approaches and identifying gaps that AI can fill. Next, prioritize areas where AI can provide the most value.
The quality and accessibility of data are paramount for effective AI implementation. Ensuring your data is clean, comprehensive, and actionable will significantly enhance the AI system’s performance.
Cross-functional collaboration among departments is essential, as risk management touches various facets of a business. Engaging various stakeholders ensures that the AI implementations address real-world challenges.
However, potential challenges include resistance to change and a lack of skilled personnel to manage AI systems. Addressing these obstacles early in the planning phase will lead to a smoother implementation process.
Pro Tip: Foster a culture of innovation and continuous learning to encourage your team to embrace AI solutions as part of their daily workflow.
Measuring Success in AI-Driven Risk Management
Defining key performance indicators (KPIs) is crucial for evaluating AI effectiveness in risk management. Some KPIs might include the accuracy of risk predictions, the speed of risk identification, and the overall reduction of risk exposure.
Continuous monitoring and adaptation of AI systems are vital; organizations should establish feedback loops to analyze performance and fine-tune algorithms regularly. This practice ensures that your AI remains relevant and effective as business conditions evolve.
Success stories abound where organizations have successfully leveraged AI, evidenced by metrics that show significant risk reduction, improved compliance, or enhanced financial outcomes. Sharing these successes can inspire confidence and commitment throughout the organization.
Pro Tip: Create dashboards that showcase real-time AI performance metrics to keep all teams informed and engaged in your risk management efforts.
The Future of AI in Risk Management
As AI technology continues to advance, emerging trends are reshaping risk management practices. Innovations like autonomous decision-making algorithms, real-time predictive modeling, and blockchain may enhance how businesses approach risk.
Regulatory changes could significantly impact how AI is utilized in risk management. Keeping abreast of these changes can help businesses remain compliant while taking advantage of AI capabilities.
Ethical implications around data privacy and AI decisions must be prioritized as well. Organizations must ensure that their AI practices do not compromise ethical standards or trust among stakeholders.
In summary, a proactive stance toward AI advancements in the risk management landscape will prepare organizations for future shifts in technology and market demands.
Pro Tip: Regular training and updates on AI trends can empower your team to harness these innovations efficiently and ethically.