DATE
1st January 2025
CATEGORY
Business Strategy, IT Operations
AUTHOR
Mirza Aditya D.
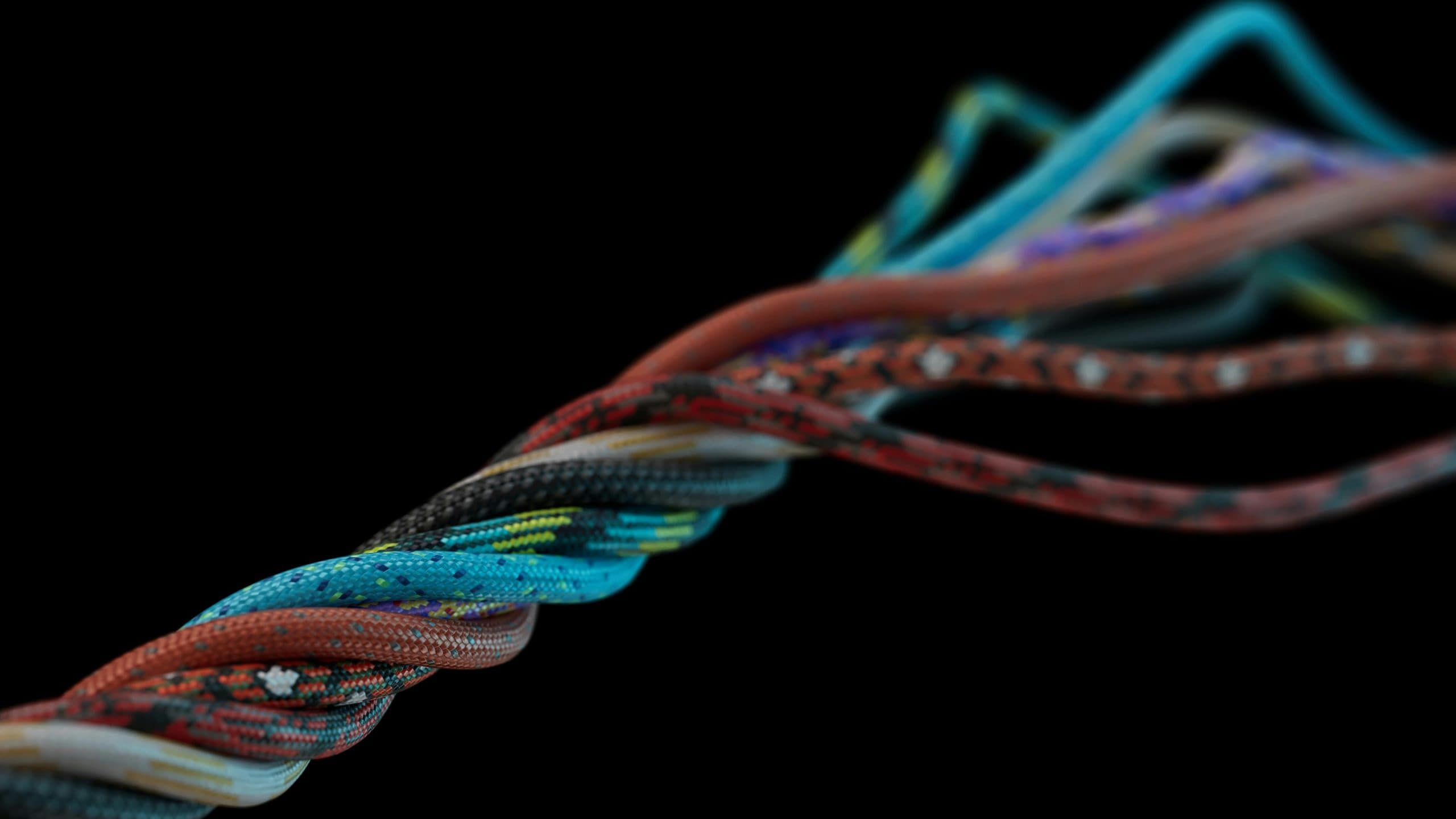
Artificial intelligence (AI) has the potential to revolutionise businesses across industries, from automating mundane tasks to unlocking unprecedented insights. Despite its big and promising potential, the path to adopting AI is fraught with challenges. Companies across various sectors face significant hurdles in integrating AI into their processes. Understanding and addressing these challenges in AI adoption is crucial to harnessing its transformative power effectively.
Lack of Data
- Challenge: AI models are data-hungry. Building robust AI systems requires access to large, high-quality, and well-labeled datasets. Many companies struggle to acquire, clean, and prepare sufficient data for their AI initiatives.
- Solutions:
- Invest in data acquisition and management: Implement data pipelines, explore data augmentation techniques, and leverage data partnerships.
- Utilise data labelling services: Employ human annotators or explore automated labelling techniques to prepare data for training.
- Focus on data quality: Ensure data accuracy, consistency, and completeness.
Skill Gap
- Challenge: A shortage of AI and data science professionals exists globally. Companies often lack the in-house expertise to develop, deploy, and maintain AI systems.
- Solutions:
- Invest in employee training and upskilling: Offer courses, workshops, and certifications to develop AI skills within the existing workforce.
- Recruit and hire AI talent: Attract and retain skilled data scientists, machine learning engineers, and AI researchers.
- Partner with academic institutions: Collaborate with universities and research institutions to access talent and gain access to cutting-edge research.
Lack of Trust and Transparency
- Challenge: Concerns around data privacy, security, and the ethical implications of AI can hinder adoption. Explaining and justifying AI decisions can be challenging, leading to a lack of trust from employees and customers.
- Solutions:
- Develop and implement robust data privacy and security measures: Ensure compliance with relevant regulations (e.g., GDPR, CCPA).
- Promote transparency and explainability: Develop AI models that are interpretable and provide clear explanations for their decisions.
- Establish ethical guidelines and principles: Ensure responsible AI development and deployment by adhering to ethical frameworks.
High Costs
- Challenge: Developing and deploying AI solutions can be expensive, involving significant investments in hardware, software, and personnel.
- Solutions:
- Explore cloud-based AI solutions: Leverage cloud platforms (e.g., AWS, Azure, Google Cloud) to access AI/ML services and infrastructure on a pay-per-use basis.
- Start with small, focused projects: Begin with pilot projects to demonstrate value and gradually scale AI initiatives.
- Consider open-source tools and frameworks: Utilise open-source technologies to reduce development costs.
Resistance to Change
- Challenge: Employees may resist the adoption of AI due to fears of job displacement, lack of understanding, and concerns about the impact on their roles.
- Solutions:
- Communicate the benefits of AI: Clearly articulate how AI can improve efficiency, productivity, and decision-making.
- Address employee concerns: Provide reassurance about job security and opportunities for reskilling and upskilling.
- Involve employees in the AI adoption process: Seek input from employees and involve them in AI-related projects.
Lack of Clear Business Objectives
- Challenge: Many companies lack a well-defined AI strategy and clear business objectives for AI adoption. Without clear goals, it is difficult to measure success and demonstrate ROI.
- Solutions:
- Define clear business objectives: Identify specific business problems that AI can solve and define measurable KPIs.
- Develop a comprehensive AI strategy: Outline the company’s vision for AI, identify key use cases, and allocate resources accordingly.
- Conduct thorough feasibility studies: Evaluate the potential impact and ROI of AI projects before implementation.
So, how’s the strategies to help us overcome the challenges for adopting AI?
Invest in Data Management
Companies must prioritise data management to ensure the success of AI initiatives. This includes implementing robust data practices, cleaning and standardizing data, and ensuring compliance with privacy regulations. Leveraging tools for data integration and quality monitoring can significantly enhance AI outcomes.
Upskilling and Training Workforce
Investing in employee training programs is essential to bridge the AI skills gap. Companies should upskill their workforce. Encouraging a culture of continuous learning can foster acceptance and enthusiasm for AI technologies.
Leverage Cloud-Based Solutions
Cloud-based AI platforms can reduce implementation costs by offering scalable and flexible solutions. These platforms eliminate the need for significant upfront investments in hardware and allow businesses to pay for resources as they use them. SMEs (Small Medium Enterprise), in particular, can benefit from the cost-efficiency of cloud-based AI.
Plan for System Integration
Effective integration of AI systems with existing infrastructure requires meticulous planning. Companies should conduct compatibility assessments, design integration roadmaps, and allocate resources for seamless implementation. Collaborating with technology partners can provide expertise and accelerate the integration process.
Adopt Ethical AI Practices
Developing ethical AI systems is paramount to building trust. Companies should implement fairness and bias mitigation techniques, ensure transparency in AI decision-making, and comply with regulatory requirements. Establishing ethics committees or guidelines can provide oversight and accountability.