DATE
23rd April 2025
CATEGORY
Artificial Intelligence, Manufacturing
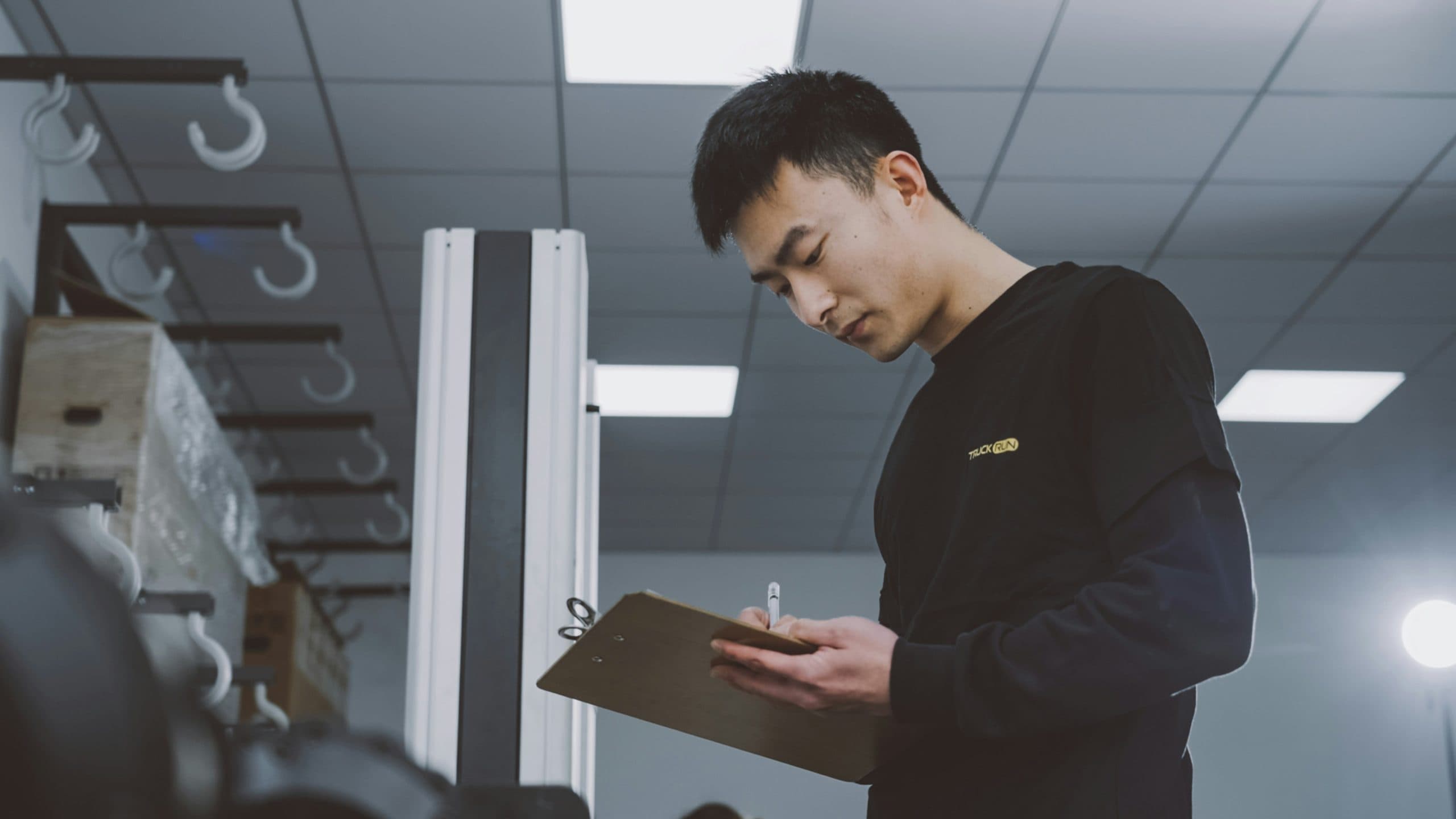
Understanding AI-Driven Quality Control in Manufacturing
AI-driven quality control refers to the application of artificial intelligence technologies to enhance the processes of ensuring product quality in manufacturing. As the industry face increasing pressures to produce high-quality products at speed, AI provides an innovative solution that is proving to be indispensable.
The critical technologies here include machine learning, computer vision, and big data analytics. Machine learning algorithms can analyze vast amounts of manufacturing data to identify patterns of defects, while computer vision technology can visually inspect products with high accuracy.
Contrasting traditional quality control methods with AI-driven approaches showcases significant differences. Traditional methods often rely on manual inspection and predefined checkpoints, whereas AI methods continuously monitor production using intelligent algorithms to catch defects in real time.
Data plays a pivotal role in AI quality control systems. By collecting and analyzing data from various points in the production process, manufacturers can develop a robust understanding of quality metrics, allowing for faster insights and improvement opportunities.
Pro Tip: Start by ensuring high-quality data collection in your manufacturing processes. Ensure data accuracy, relevance, and timeliness to fully leverage AI capabilities.
Benefits of Implementing AI in Quality Control
The advantages of incorporating AI into quality control are numerous. First and foremost, AI significantly improves defect detection rates and reduces false positives. This means that manufacturers can produce higher-quality outputs without the worry of sending faulty products to market.
Additionally, AI enhances production efficiency by minimizing downtime. Algorithms can predict when machinery is likely to fail or when a production line is nearing a bottleneck, allowing for timely interventions.
Real-time data analysis is another significant advantage. With AI, you can access insights instantly, facilitating immediate decision-making that optimizes production.
Cost reduction is also a notable benefit. With AI systems cutting waste and rework, manufacturing companies can save money and allocate resources more effectively.
Finally, AI fosters continuous improvement through predictive analytics. By learning over time, these systems can continuously enhance their processes based on historical data and trends.
Pro Tip: Regularly review the impacts and changes brought about by AI on your quality control processes, and be ready to adapt as needed to maximize the benefits.
Key Components of an AI Quality Control System
An effective AI quality control system comprises several key components. Relevant hardware includes sensors, cameras, and other devices that capture various metrics during production.
Equally important is the software that utilizes AI algorithms to analyze this data. It is vital to invest in robust AI platforms capable of sophisticated analysis to ensure quality metrics are met.
Data management systems are essential for tracking and managing quality metrics. They provide a foundation for effective decision-making by collating necessary data in one place.
The choice between edge computing and cloud solutions can significantly impact your organization’s real-time processing capabilities. Edge computing can process data on-site, reducing latency, while cloud solutions provide greater flexibility and scalability.
Finally, integration with existing manufacturing processes is crucial. Ensure your AI system can seamlessly connect with current operations to minimize disruption.
Pro Tip: Assess existing processes and identify areas where AI can provide immediate value to prioritize integration efforts and resources.
Real-World Applications and Case Studies
A notable case study includes a leading electronics manufacturer that employed AI technology for visual inspection, resulting in a 30% improvement in defect detection rates. Such implementations have illuminated pathways for effective quality assurance through AI.
Lessons learned from these successful projects emphasize the importance of employee buy-in and proper training. What’s more, these cases highlight the potential for AI applications to grow in other sectors of manufacturing.
Pro Tip: Stay informed about advancements in AI applications from industry peers, as learning from others can provide a clear roadmap for implementation in your own processes.
Challenges and Considerations in Implementation
Initial costs and investments can deter companies from proceeding. However, it is essential to view AI as a long-term investment that can yield substantial returns over time.
Employee training and skill development are critical. Without proper training, staff may struggle to utilize new technologies effectively, inhibiting the success of AI systems.
Data privacy and cybersecurity concerns must also be prioritized, as sensitive information may be at risk during the implementation of AI systems.
Pro Tip: Develop a clear communication plan about the benefits and support available to your team during the transition to AI-driven quality control.
Steps to Implement AI-Driven Quality Control
Next, identify suitable AI technologies that align with your business goals. Research different solutions that fit your production environment and quality metrics needs.
Developing a pilot program is crucial. Testing AI solutions on a smaller scale allows you to identify potential challenges before full implementation.
Establish key performance indicators (KPIs) to measure success. These metrics will help assess the effectiveness of AI implementations and inform decision-making moving forward.
Lastly, fostering a culture of innovation and continuous feedback is key. Encourage employees to share insights, ideas for improvement, and experiences with the new systems.
Pro Tip: Ensure your pilot program is highly visible within the organization. Share successes and learnings widely to gain further buy-in from stakeholders.