DATE
2nd April 2025
CATEGORY
Artificial Intelligence, Business Strategy, IT Operations
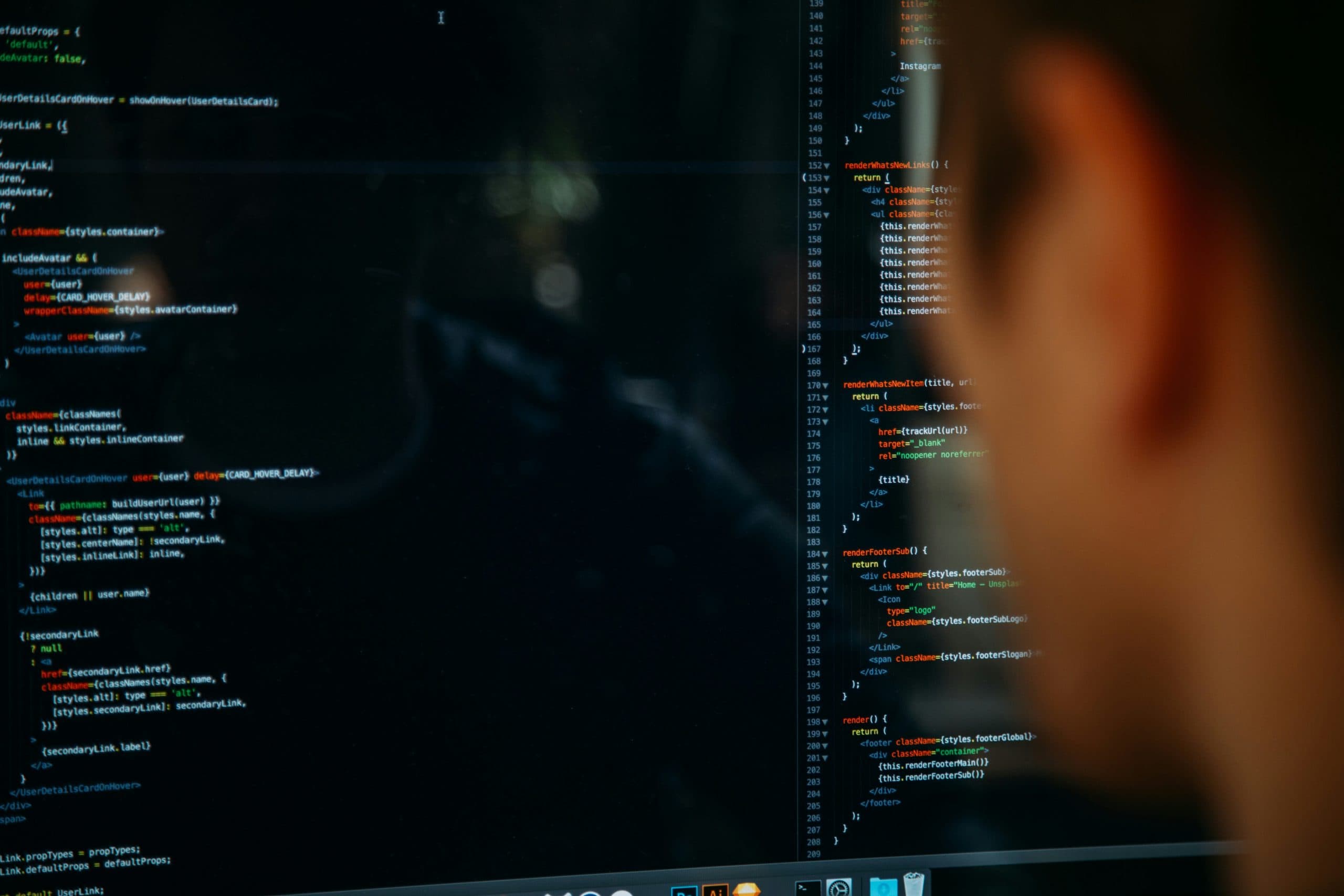
Introduction to Machine Learning in Business
Machine learning (ML) has emerged as a pivotal component in modern business practices, transforming how organizations operate, make decisions, and serve customers. At its core, machine learning is a subset of artificial intelligence that enables systems to learn from data, identify patterns, and make decisions with minimal human intervention.
In today’s data-driven landscape, executives and middle-level managers find themselves increasingly reliant on actionable insights derived from vast volumes of data. Companies that harness machine learning enhance their decision-making capabilities, leading to more effective strategies and improved outcomes.
The potential for machine learning to elevate operational efficiency is immense. By automating repetitive tasks, companies can reduce costs and redirect resources toward innovation and growth. Moreover, integrating ML into workflows can streamline operations, enabling organizations to remain agile in a competitive climate.
In summary, machine learning can transform operational workflows, empowering businesses to respond proactively to market demands and drive continuous improvement.
Types of Machine Learning Algorithms
Understanding the various types of machine learning algorithms is crucial for pinpointing the best applications for business needs.
Supervised learning algorithms utilize labeled datasets to make predictions. Common applications in business include credit scoring and email filtering. For instance, companies can use logistic regression to predict customer churn based on historical interaction data.
Unsupervised learning algorithms analyze data without pre-existing labels, effectively uncovering hidden patterns. This is particularly beneficial for market segmentation analysis. For example, clustering methods like K-means can help businesses understand distinct customer groups for targeted marketing.
Reinforcement learning is particularly suited for dynamic environments where systems learn through trial and error. Its applications include inventory management and dynamic pricing. A well-known example is Google’s use of reinforcement learning to optimize its data center operations.
Pro Tip: Always begin the implementation of ML algorithms with a clear understanding of the desired outcome to ensure alignment with business objectives.
Benefits of Implementing Machine Learning Algorithms
The practical benefits of incorporating machine learning algorithms into business operations are numerous.
One of the most significant advantages is streamlining processes through automation. Repetitive tasks, such as data entry or report generation, can be delegated to machines, freeing up valuable employee time for core, strategic initiatives.
Moreover, predictive analytics enhances decision-making by providing insights based on historical data. For example, retailers can analyze purchasing trends to anticipate customer needs, thereby improving inventory management.
Customer experience also benefits from machine learning. By personalizing services and communications, businesses can engage customers more effectively, resulting in higher satisfaction rates and improved loyalty.
Finally, optimizing resource allocation using ML leads to elevated operational efficiency. Businesses can intelligently allocate resources, ensuring that they are used where they provide the most value.
Key Steps to Integrate Machine Learning into Business Operations
Integrating machine learning into business operations requires a strategic approach.
Start by conducting a thorough assessment of existing operational processes. Understanding your current state will help identify areas ripe for improvement.
Next, pinpoint specific business challenges that machine learning can address. This could range from optimizing logistics to enhancing customer support.
Collaboration with data scientists or machine learning experts is vital. They can guide you in choosing appropriate algorithms tailored to your identified problem areas.
Finally, develop a roadmap for implementation, including necessary training for staff and securing resources, to ensure a smooth transition to machine learning-enhanced operations.
Real-world Case Studies: Success Stories
Examining real-world case studies of organizations that have successfully integrated machine learning provides valuable insights.
For instance, Amazon has utilized machine learning to streamline its supply chain, analyzing demand patterns to optimize inventory levels. The result? Significant cost savings and improved delivery times.
Another example is Netflix, which uses machine learning for content recommendation. By analyzing viewer preferences, they increase user engagement, leading to higher subscription retention rates.
However, these journeys are not without challenges. Many organizations face data quality issues and resistance to change during implementation. Leadership plays a crucial role in championing ML initiatives and fostering a culture of acceptance.
Challenges and Considerations in Machine Learning Adoption
Despite its myriad benefits, the adoption of machine learning also presents several challenges.
Data privacy and security remain paramount concerns. Ensuring that data handling complies with regulations is crucial to building customer trust.
Another consideration is the quality of data. Clean datasets are essential for effective machine learning outcomes. Companies must invest in data hygiene to avoid misleading predictions.
Resistance to change can also impede progress. Employees might view ML as a threat, necessitating clear communication about its role as a tool for enhancement rather than replacement.
Finally, ongoing training and technological updates are necessary to keep pace with advancements in machine learning methodologies.
Future Trends in Machine Learning and Business Operations
The landscape of machine learning in business is continuously evolving.
Emerging technologies, such as natural language processing (NLP) and computer vision, are driving innovation and expanding the scope of applications. For example, voice-activated assistants are being integrated into customer service frameworks.
Ethics in AI will also shape future machine learning applications, pressing businesses to consider the implications of automated decision-making on society and individual rights.
As customer expectations rise, businesses must adapt by leveraging machine learning to deliver enhanced, personalized experiences.
Finally, the potential for increased collaboration between humans and machines will redefine workplace dynamics, fostering a symbiotic relationship that leverages the strengths of both.
To sum up, embracing machine learning is no longer optional for organizations aiming for operational excellence. The insights and efficiencies gained from ML integration can position them ahead of the competition.
Executives and managers are encouraged to start small, testing and scaling successful implementations. This approach minimizes risk while fostering a culture of innovation.
Reflecting on your own organization’s operations can reveal numerous opportunities for machine learning integration. What processes can be streamlined? How can decision-making be enhanced?
For further reading and professional development in machine learning, consider exploring reputable online courses or subscribing to industry-leading publications. Embracing this technology is the first step towards redefining your business’s future.