DATE
6th April 2025
CATEGORY
Artificial Intelligence, Business Strategy, Strategy Management
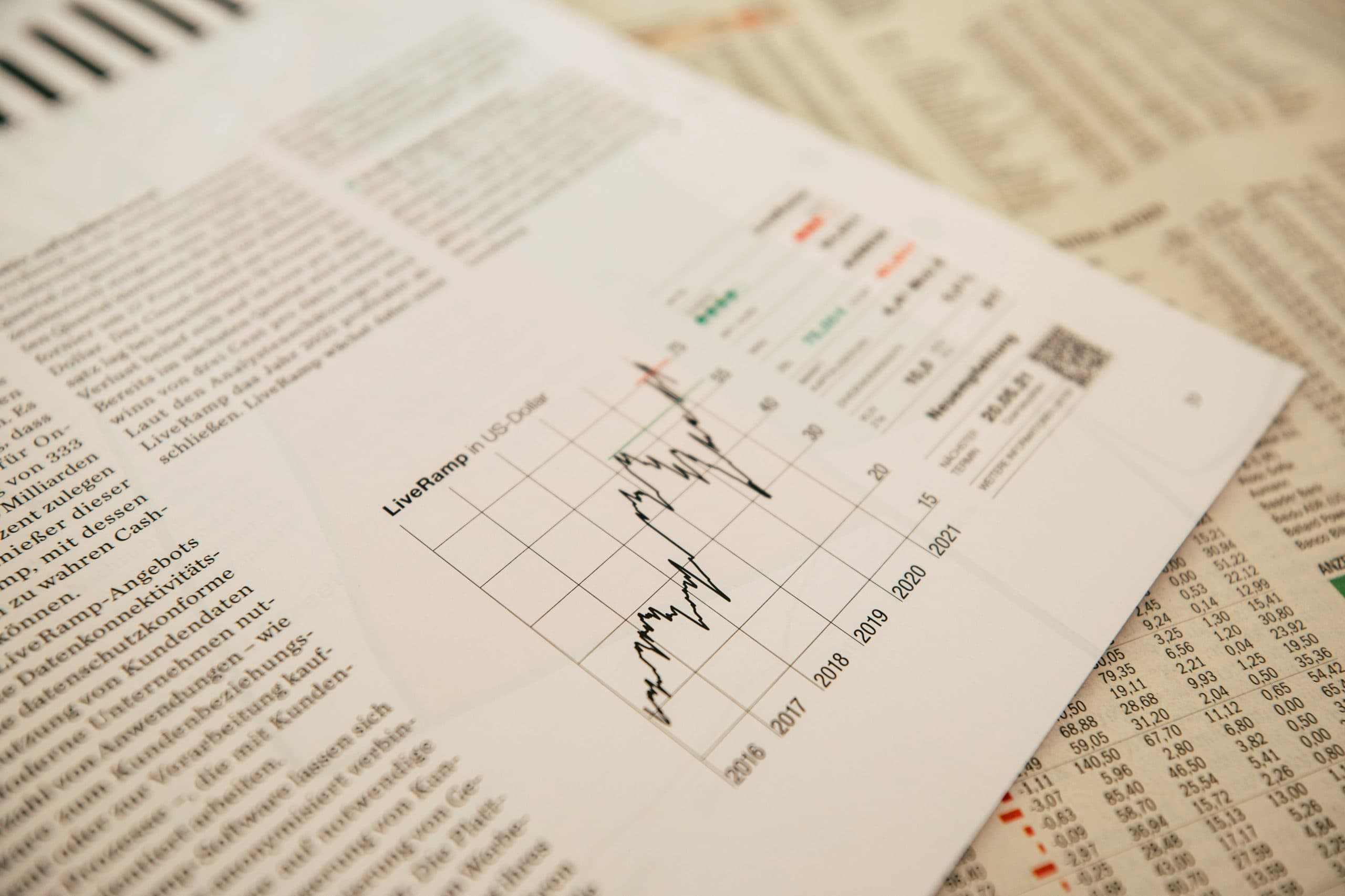
Understanding Predictive Analytics: An Overview
Predictive analytics is a branch of advanced analytics that uses statistical algorithms and machine learning techniques to identify the likelihood of future outcomes based on historical data. The core components include data mining, predictive modeling, and machine learning.
The evolution of predictive analytics in business dates back to when companies first began using statistical methods to make forecasts. Today, advancements in technology, especially the rise of big data, have accelerated this field, transforming it into a powerful tool for strategic decisions.
Common tools and technologies utilized in predictive analytics include platforms like R, Python, SAS, and machine learning libraries such as TensorFlow. These tools help interpret large datasets and generate actionable insights.
Unlike traditional analytics, which primarily focuses on explaining historical performance, predictive analytics allows businesses to anticipate future trends, providing a proactive rather than reactive approach to decision-making.
Pro Tip: For those new to predictive analytics, start with an accessible tool like Google Analytics, which offers basic predictive insights to familiar yourself with the concepts before diving into more complex systems.
The Importance of Predictive Analytics
Predictive analytics can significantly enhance forecasting and strategic planning by providing more accurate projections of future events. For instance, businesses can anticipate customer purchasing behavior, leading to better inventory management.
In risk management, predictive analytics for business can proactively identify potential risks and devise appropriate mitigation strategies. For example, financial institutions utilize predictive models to minimize loan defaults by assessing customer creditworthiness more effectively.
Case studies show how industries like retail have successfully implemented predictive analytics. For instance, Target’s use of shopping data analytics to predict pregnancy among customers has revolutionized their marketing strategies, proving the power of data-driven decisions.
Gaining a competitive advantage through predictive analytics isn’t just about numbers; it’s about making informed decisions that drive innovation and improve customer satisfaction.
Pro Tip: To understand the value of predictive analytics better, conduct small-scale pilot projects within your organization. This way, you can glean insights from actual data without investing heavily upfront.
Key Steps to Integrate Predictive Analytics
The first step in adopting predictive analytics for business is assessing your organization’s readiness. Understanding your infrastructure, culture, and overall data literacy among staff can help you gauge how prepared you are for this transition.
Identifying key data sources and ensuring their quality is vital. This involves cleaning up existing data and integrating new data sources to create a robust dataset that supports predictive modeling.
Create a cross-functional team that includes data scientists, subject matter experts, and IT personnel to drive analytics initiatives effectively. Collaboration ensures alignment on goals and enhances the implementation process.
Establishing clear objectives and KPIs for your predictive analytics projects helps track progress and measure success. Ensure that these objectives align with your overall business strategy.
Pro Tip: Use a phased approach to implementation. Start with one department or function, assess outcomes, and expand gradually based on successes and insights gained.
Challenges in Utilizing Predictive Analytics
Common roadblocks in implementing predictive analytics include data silos, where different departments hoard data and prevent effective collaboration. This requires a cultural shift emphasizing data sharing and transparency.
Addressing the skills gap is also crucial; many organizations face a shortage of personnel skilled in analytics. Investing in training and development can help bridge this gap.
Data privacy and ethical considerations must not be neglected. Businesses must ensure that their use of predictive analytics complies with regulations such as GDPR and fosters trust with consumers.
Integration challenges with legacy systems can hinder the adoption of predictive analytics. It’s crucial to invest in the right technology that aligns with your current systems to ease the transition.
Pro Tip: Regularly conduct stakeholder reviews to identify and address any obstacles as they arise. This proactive approach ensures that your analytics strategy remains aligned with business goals.
Real-world Applications
Predictive analytics has a wealth of use cases across various sectors. In retail, businesses employ predictive analytics for inventory optimization, allowing for better stock management and increased sales.
In healthcare, predictive maintenance can prevent equipment failures, ensuring operational efficiency and improved patient care. By analyzing past maintenance data, hospitals can foresee and address potential issues before they arise.
Customer segmentation and targeted marketing are other powerful applications. Analyzing customer data enables companies to tailor their marketing efforts, significantly improving conversion rates.
In workforce optimization, predictive analytics can help businesses forecast staffing needs based on historical data trends, ensuring they have the right number of employees for peak operational times.
Pro Tip: To maximize the benefits of predictive analytics, regularly review and refine models based on new data to ensure continued accuracy and relevance to current market conditions.
Measuring the Success
Defining metrics for evaluating the success of your predictive analytics efforts is essential. Common KPIs include accuracy of predictions, ROI from initiatives, and improved operational efficiency.
Feedback loops and continuous improvement are crucial. By regularly assessing the accuracy of predictions and the efficacy of actions taken based on insights, businesses can adapt and refine their strategy over time.
Share case studies illustrating measurable business outcomes; these real-life examples can motivate your team and stakeholders by showcasing successful applications of predictive analytics.
Leverage key learnings to ensure the long-term success of analytics initiatives. Understanding what worked and what didn’t allows for a more robust implementation strategy in the future.
Pro Tip: Develop a comprehensive reporting system that tracks performance metrics related to predictive analytics initiatives. Regular updates can help keep stakeholders informed and engaged in the process.
Future Trends in Predictive Analytics
The impact of AI and machine learning on predictive analytics will only deepen. As these technologies evolve, they enhance predictive models, enabling even more accurate forecasts and insights.
Real-time analytics is on the rise, allowing businesses to make immediate decisions based on current data—a game changer for industries that rely on speed and agility.
Integration with IoT and big data will also transform the landscape. As more devices collect data, businesses can harness this information for more granular and timely insights.
In the future, the demand for skills and competencies in analytics-driven roles will rise. Organizations should prepare their workforce by investing in training tailored to the evolving landscape of predictive analytics.
Pro Tip: Stay ahead by engaging with industry thought leaders and attending webinars or conferences focused on predictive analytics. Networking and learning from shared experiences can foster innovative ideas for your organization.